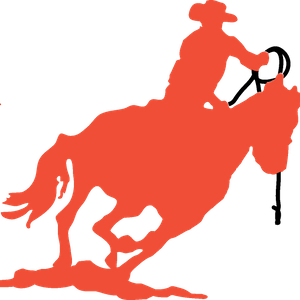
DATA SCIENCE, AI & MACHINE LEARNING CONSULTING
ABOUT
Data Cowboys is a boutique data science and machine learning consulting cooperative, offering custom expert-level solutions for complex data problems. We have over a decade of academic and real-world experience in turning challenges into practical algorithms using a battery of machine learning, artificial intelligence, data science, and statistics tools, and we take pride in clearly communicating our results to audiences of all backgrounds.
Services provided include applications of deep learning and other AI techniques (convolutional networks, transformers, large language models, adversarial training, etc) to problems in computer vision, natural language processing, decision-making under uncertainty, chatbots, and other applications. With advanced techniques and expert insights, we have consistently succeeded in using, controlling and optimizing large language models such as OpenAI's ChatGPT and Anthropic's Claude to meet our diverse application demands.
Blog
Although "big data" and "deep learning" are dominant, my own work at the Gates Foundation involves a lot of small (but expensive) datasets, where the number of rows (subjects, samples) is between 100 and 1000. For example, detailed measurements throughout a pregnancy and subsequent neonatal...November 16, 2020Note: this blog post is an expanded version of my recent Twitter thread. A paper was posted to arXiv this November that gives name to a phenomenon that I've had plenty of experience with, but never had a word for. From the abstract: "An ML pipeline is underspecified when it can return many...October 26, 2020Note: this blog post first appeared elsewhere and is reproduced here in a slightly altered format. 2020 is the year of search for Semantic Scholar (S2), a free, AI-powered research tool for scientific literature, based at the Allen Institute for AI. One of S2's biggest endeavors this year is to...TESTIMONIALS
"We've really enjoyed working with Data Cowboys. I have explained our startup to hundreds of people and to this day Sergey and Ilya grasped what we do the fastest. Within minutes, our first conversation transitioned from context to idea generation, and it has been the same for every meeting since. They are professional, timely, and have a great sense of humor to boot. And they are so fast - both with their thinking and their output! Thanks to their efforts we have not yet had to hire data scientists in-house, saving us lots of money as well."
Jay Goyal, Co-Founder, Actively Learn
"Sergey's machine learning consulting expertise has been invaluable to us at RichRelevance. He has a wonderful knack for quickly scoping a problem, alighting on a suitable machine learning solution, making efficient and early decisions on model choice and parameters, analyzing data, implementing solutions, and clearly communicating throughout the process. His solid grasp of Bayesian statistics, deep neural networks, online learning and optimization etc, and his ready and speedy application have produced significant and concrete value for us on many occasions."
Apu Mishra, Lead Data Scientist, RichRelevance
"Sergey has helped me with the writing of two books about data analytics: Data Driven and The Data Driven Leader. He's easy to work with, and provides well-considered and well-written results very quickly. While helping me with the technical aspects of my books, he consistently exhibited in-depth knowledge of analytics, machine learning, and data science, and he has the rare ability to communicate that knowledge clearly to readers at every level. I would be happy to recommend him to anyone requiring machine learning services of any kind."
Jenny Dearborn, Chief People Officer, Klaviyo
"Sergey Feldman is the ideal combination you want in a consultant: he’s brilliant, knows his subject matter inside and out, and is great to work with – collaborative, candid and funny. He’s also typically lightning-fast, a straight shooter and accepts feedback readily and with grace. He also has a special gift for explaining complex analytical and other concepts in terms anyone can understand. I’d hire him again in a heartbeat, with total confidence that he will shine, get the job done right the first time and make me look great."
Deb Arnold, Principal at Deb Arnold, Ink.
"The work Data Cowboys has done to support our anti-trafficking efforts has been incredible. We are not only able to confidently understand and share the data generated by the work that we do, we're also able to verify that we're having the impact we intend - to keep people safe from exploitation. Sergey is responsive, thoughtful, and a tremendous asset to our mission."
Robert Beiser, Strategic Initiatives Director for Sex Trafficking, Polaris
Tell us about your data challenges.
ILYA@DATA-COWBOYS.COM